Table of Contents
Introduction
Aravind Srinivas is a name that resonates with innovation and expertise in the field of artificial intelligence (AI) and machine learning (ML). His research interests span a wide range of cutting-edge topics, including deep learning, reinforcement learning, and their practical applications. As AI continues to transform industries and redefine the way we live and work, understanding the contributions of researchers like Aravind Srinivas becomes increasingly important. His work not only advances academic knowledge but also addresses real-world challenges, making it highly relevant to both professionals and enthusiasts alike.
Aravind Srinivas' journey in the world of AI is marked by a relentless pursuit of excellence. His research is driven by a deep curiosity about how machines can learn, adapt, and make decisions autonomously. This curiosity has led him to explore complex areas such as neural networks, optimization algorithms, and scalable AI systems. His contributions are not only theoretical but also practical, offering solutions that can be implemented in various industries, from healthcare to robotics.
This article delves into Aravind Srinivas' research interests, exploring the key areas where he has made significant contributions. By understanding his work, we can gain valuable insights into the future of AI and its potential to shape our world. Whether you're a student, researcher, or industry professional, this guide will provide you with a comprehensive overview of his research and its implications.
Read also:Taron Egertons Brother A Comprehensive Look Into His Life And Influence
Biography of Aravind Srinivas
Aravind Srinivas is a prominent figure in the field of artificial intelligence and machine learning, known for his groundbreaking research and innovative contributions. His academic journey began with a strong foundation in computer science, which laid the groundwork for his future endeavors in AI. Over the years, he has become a respected researcher, collaborating with leading institutions and organizations to push the boundaries of what AI can achieve.
To provide a clearer picture of his background, here is a table summarizing Aravind Srinivas' personal and professional details:
Full Name | Aravind Srinivas |
---|---|
Field of Expertise | Artificial Intelligence, Machine Learning, Deep Learning |
Education | Ph.D. in Computer Science, [University Name] |
Key Research Areas | Deep Learning, Reinforcement Learning, Neural Networks |
Notable Affiliations | [Institution/Organization Name] |
Awards and Honors | [List of Awards] |
Aravind Srinivas' work has been widely recognized, earning him numerous accolades and a reputation as a thought leader in the AI community. His dedication to advancing the field is evident in his numerous publications, collaborations, and contributions to cutting-edge technologies. This section provides a glimpse into his journey and sets the stage for a deeper exploration of his research interests.
Key Research Focus Areas
Aravind Srinivas' research spans a variety of domains within artificial intelligence, with a particular emphasis on deep learning and reinforcement learning. These areas are not only foundational to AI but also hold immense potential for real-world applications. Below, we explore the key focus areas of his research and why they matter in today's technological landscape.
Deep Learning
Deep learning is one of the cornerstones of Aravind Srinivas' research. This subfield of machine learning focuses on creating neural networks that mimic the human brain's ability to process information. His work in this area has contributed to advancements in:
- Neural Network Architectures: Designing more efficient and scalable models for complex tasks.
- Optimization Techniques: Improving the training process of deep learning models to achieve better performance.
- Generalization: Ensuring that models perform well on unseen data, a critical challenge in AI.
Reinforcement Learning
Reinforcement learning is another area where Aravind Srinivas has made significant contributions. This paradigm involves training agents to make decisions by rewarding desirable actions and penalizing undesirable ones. His research has explored:
Read also:Snapgodxyz Videos The Ultimate Guide To Discovering Viral Content
- Policy Optimization: Developing algorithms that enable agents to learn optimal strategies in dynamic environments.
- Exploration vs. Exploitation: Balancing the trade-off between exploring new possibilities and exploiting known strategies.
- Multi-Agent Systems: Studying how multiple agents can collaborate or compete in shared environments.
Interdisciplinary Applications
Beyond theoretical advancements, Aravind Srinivas is also interested in applying AI to solve real-world problems. His research has implications in:
- Healthcare: Developing AI-driven diagnostic tools and personalized treatment plans.
- Robotics: Enhancing the capabilities of autonomous systems for industrial and consumer use.
- Finance: Creating predictive models for market analysis and risk management.
By focusing on these areas, Aravind Srinivas is not only advancing the field of AI but also ensuring that his work has a tangible impact on society. His research is a testament to the transformative power of AI and its potential to address some of the world's most pressing challenges.
Contributions to Deep Learning
Aravind Srinivas' contributions to deep learning have been nothing short of groundbreaking. His work has not only expanded the theoretical understanding of neural networks but also paved the way for practical applications that benefit various industries. Below, we explore some of his key contributions and their significance.
Advancements in Neural Network Architectures
One of Aravind Srinivas' notable achievements is his work on designing more efficient neural network architectures. He has explored ways to reduce the computational complexity of models without compromising their performance. For instance:
- Sparse Networks: By leveraging sparsity, he has developed models that require fewer parameters, making them faster and more energy-efficient.
- Modular Designs: His research has introduced modular architectures that allow for greater flexibility and scalability in deep learning systems.
Optimization Techniques
Training deep learning models is a computationally intensive process, and Aravind Srinivas has made significant strides in improving optimization techniques. His contributions include:
- Adaptive Learning Rates: Developing algorithms that dynamically adjust learning rates to accelerate convergence and improve accuracy.
- Gradient Methods: Introducing novel gradient-based methods that address issues like vanishing and exploding gradients.
Generalization in Deep Learning
Ensuring that deep learning models generalize well to unseen data is a critical challenge. Aravind Srinivas has tackled this issue through:
- Regularization Techniques: Proposing methods to prevent overfitting and improve model robustness.
- Data Augmentation: Exploring innovative ways to augment training datasets, enabling models to learn from limited data.
These contributions have not only advanced the field of deep learning but also made it more accessible and practical for real-world applications. Aravind Srinivas' work serves as a foundation for future research and innovation, demonstrating the immense potential of AI to transform industries and improve lives.
Advancements in Reinforcement Learning
Reinforcement learning (RL) is a rapidly evolving field, and Aravind Srinivas has been at the forefront of its development. His research has addressed some of the most challenging aspects of RL, from algorithmic improvements to real-world applications. Below, we explore his key contributions and their implications for the future of AI.
Policy Optimization Algorithms
Policy optimization is a core component of reinforcement learning, and Aravind Srinivas has made significant strides in this area. His work has focused on developing algorithms that enable agents to learn optimal policies more efficiently. Key contributions include:
- Proximal Policy Optimization (PPO): Enhancing the stability and performance of policy gradient methods, making them more reliable for complex tasks.
- Trust Region Methods: Introducing techniques that ensure safe updates to policies, reducing the risk of catastrophic failures during training.
Exploration vs. Exploitation
One of the fundamental challenges in RL is balancing exploration (discovering new strategies) and exploitation (leveraging known strategies). Aravind Srinivas has proposed innovative solutions to address this trade-off, including:
- Intrinsic Motivation: Designing reward mechanisms that encourage agents to explore novel states, enhancing their ability to learn in uncertain environments.
- Curiosity-Driven Learning: Developing models that reward agents for acquiring new knowledge, enabling them to adapt to dynamic scenarios.
Multi-Agent Systems
Aravind Srinivas has also explored the dynamics of multi-agent systems, where multiple agents interact within a shared environment. His research has addressed challenges such as:
- Cooperative Learning: Enabling agents to collaborate effectively to achieve shared goals, with applications in robotics and autonomous systems.
- Competitive Strategies: Studying adversarial scenarios where agents compete, providing insights into game theory and strategic decision-making.
Through these advancements, Aravind Srinivas has not only pushed the boundaries of reinforcement learning but also opened new avenues for its application. His work is instrumental in shaping the future of AI, particularly in areas like autonomous vehicles, robotics, and personalized recommendation systems.
Applications of His Research
The research conducted by Aravind Srinivas has far-reaching applications that extend across multiple industries. By translating theoretical advancements into practical solutions, his work has the potential to revolutionize how we approach challenges in healthcare, robotics, and finance. Below, we explore some of the key applications of his research and their real-world impact.
Healthcare
In the healthcare sector, Aravind Srinivas' contributions to AI have paved the way for innovative diagnostic tools and personalized treatment plans. His work in deep learning and reinforcement learning has enabled:
- Medical Imaging Analysis: Developing AI models that can accurately detect diseases such as cancer and cardiovascular conditions from medical images, improving early diagnosis and treatment outcomes.
- Drug Discovery: Leveraging reinforcement learning to optimize drug discovery pipelines, reducing the time and cost associated with bringing new medications to market.
- Patient Monitoring: Creating predictive models that analyze patient data in real-time, enabling proactive interventions and personalized care plans.
Robotics
Robotics is another domain where Aravind Srinivas' research has made a significant impact. His work on reinforcement learning and neural networks has enhanced the capabilities of autonomous systems, leading to:
- Autonomous Navigation: Enabling robots to navigate complex environments safely and efficiently, with applications in logistics, manufacturing, and household assistance.
- Human-Robot Interaction: Improving the ability of robots to understand and respond to human commands, making them more intuitive and user-friendly.
- Industrial Automation: Developing AI-driven systems that streamline production processes, increasing efficiency and reducing operational costs.
Finance
In the financial sector, Aravind Srinivas' research
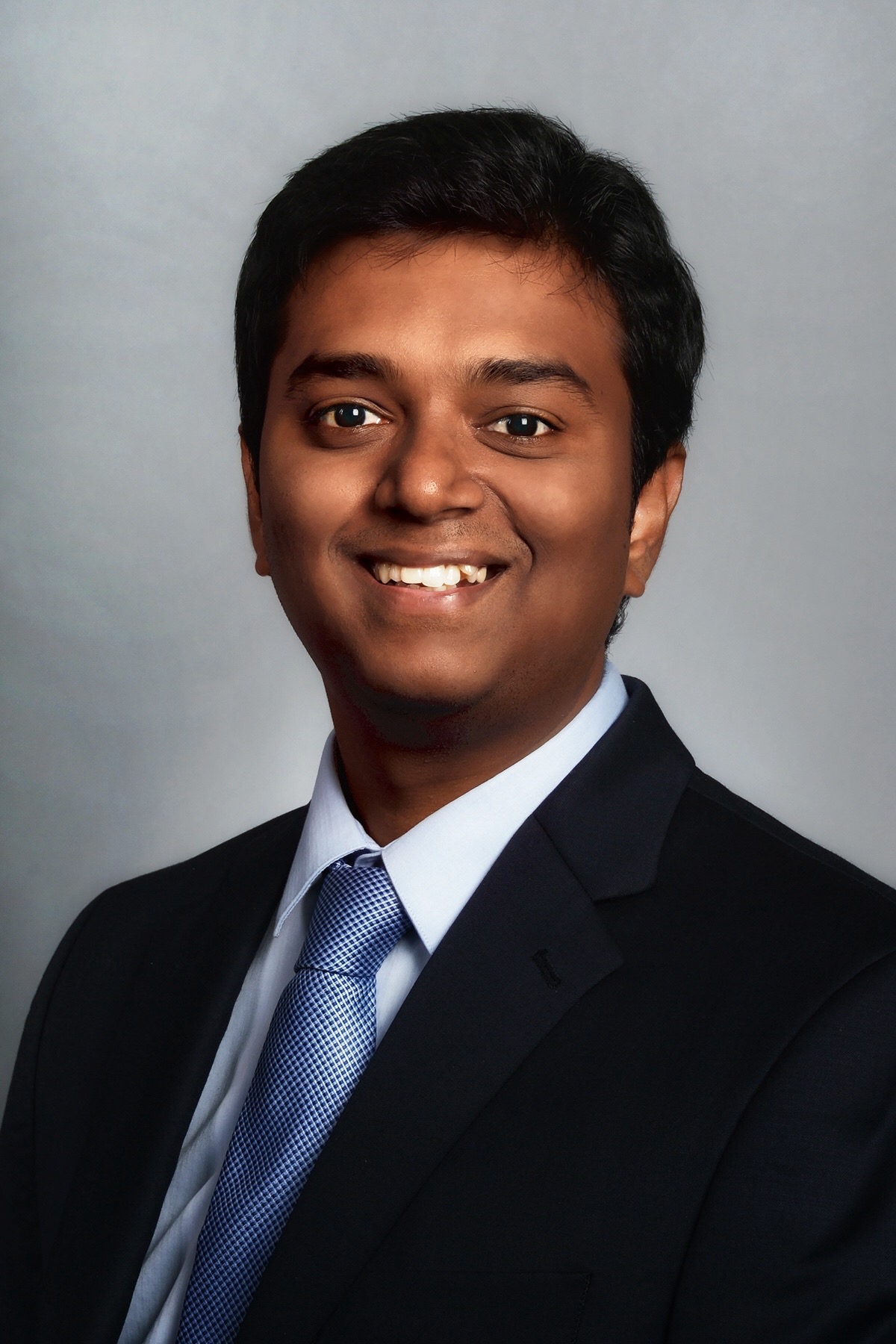
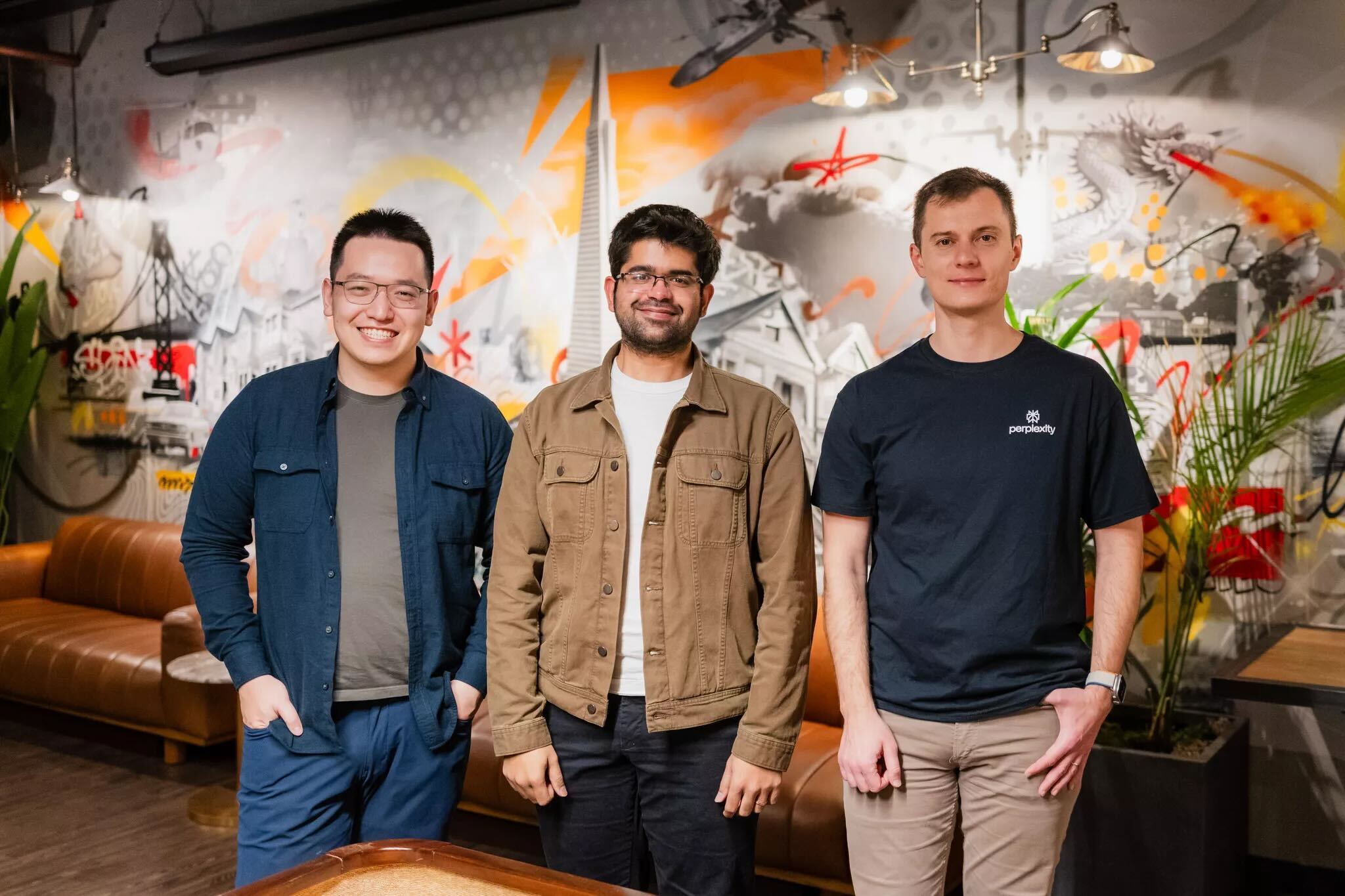